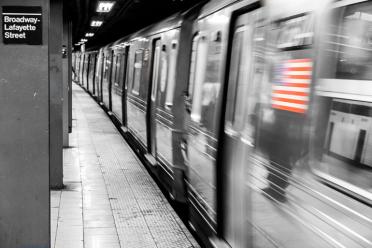
As the review states in the first line of the conclusion: “Assembling genomes out of heterogeneous samples is an extremely challenging problem and one that remains unsolved.” Indeed, with the first specialised tools for dedicated assembly of metagenomic data only released in 2012, the field is still in its infancy.
Yet, metagenomics is a rapidly expanding field of research, with some important implications in healthcare, especially. Gleaning accurate information on the contents of the human gut, for example, can help us move forward the area of personal healthcare and more swift diagnosis of life-threatening conditions.
Likewise, analysing environmental samples - envirogenomics - can help us explore the composition of the complex communities of microorganisms living all around us, even on and inside us: the human microbiome, the New York Subway, the virome of bats, the oceans, the crop rhizosphere and the extremophiles living in hot springs, to name but a few already-applied examples.
Additionally, metagenomics can provide a means to investigate species which are otherwise impossible to interrogate using classic genomics methods.
The challenges are abundant in such analyses, however. Firstly, there is unknown abundance and diversity in any sample. How to know how much is in there, and how much of what? It’s not possible to make many of the assumptions that you could with regard to samples gleaned from a single species, when it comes to assembly of the sequence.
Another problem is related species: “in a genomic study, it may be assumed that all sequence reads derive from the same original genome. In metagenomic studies, this is emphatically not the case.” In a metagenomic sample, there are so many related species and subspecies that these can really confuse an assembler - with extensive overlaps in a kmer set, for example.
Other significant challenges include memory and processing, initial classification of reads, graph partitioning and read pair information.
So, what are the approaches? Find out in the review - in which the team looks at a variety of short-read de novo tools, as well as looking at reference-based approaches. Currently there are no published tools dedicated solely to metagenomes gleaned from third generation, long-read sequencing platforms, but good results have yet been garnered from the use of existing tools.
Essentially, assembling metagenomes requires different algorithmic approaches, which don’t abide by the assumptions made by most genome assembly tools. New tools are continuing to emerge, but there is no single tool that is best for all samples or questions.